-
Notifications
You must be signed in to change notification settings - Fork 94
New issue
Have a question about this project? Sign up for a free GitHub account to open an issue and contact its maintainers and the community.
By clicking “Sign up for GitHub”, you agree to our terms of service and privacy statement. We’ll occasionally send you account related emails.
Already on GitHub? Sign in to your account
--multi-class not splitting images with multiple boxes correctly #12
Comments
Looks like that was an incomplete fix. With that, any images that have multiple boxes in the validation set are created correctly there, but they will also be created in the training set. The problem seems to be that it's splitting the annotations properly by class, but doesn't take into account that some of those split annotations are in the same file. |
I'm sure there's a better way actually using iterative_train_test_split, but here is my quick and dirty fix. It gets all the files which were set as validation and removes them from the training set before grabbing all their annotations. This does mean the split won't be as requested and will actually be very different between the two modes. This gives priority to the test set if args.multi_class:
|
@fama0 This seems to work well, thanks! |
I would like to use the --multi-class option, but I noticed that it would only split the image and the first bounding box declaration into the validation set. If the image had more boxes, those would be left orphaned on the training set file. Removing the flag allows that same image to have all it's box definitions in the validation set. I checked that this same bug is in the ahmad-ra repository so it's not something that came up due to the merge
I don't know if this is the best way of fixing it, but I took the working code as an example and modified the multi-class specific one to filter_annotations in hopes of getting all the annotations for the image. That did work
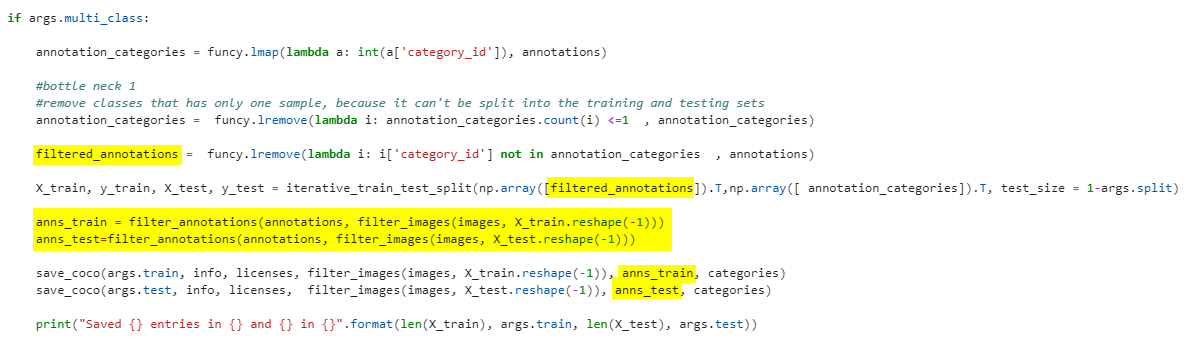
The text was updated successfully, but these errors were encountered: